This project seeks to automate aspects of the learning disability detection process using machine learning, with the goal of identifying characteristics in children’s handwriting and placing them in the diagnostic queue sooner, increasing their chances of high school graduation and lifelong literacy.
How does it work?
Why does it matter?
Dyslexia Fast Facts
- It is estimated that up to 20% of students in the U.S. have dyslexia or another language-based learning disability1 — that’s 11.32 million kids
- If a child struggles to read in third grade they are four times more likely to drop out before graduating from high school2
- People with low literacy or numeracy skills are up to eight times more likely to be unemployed. Those who are employed are likely to be low-paid3. This is after accounting for the socioeconomic status of their upbringing
- Teachers are not trained to detect dyslexia4. In fact, 33% of educators say that what people call a learning or attention issue is just laziness1
- In many states, the word dyslexia is not properly defined, allowing districts to delay diagnosis
Background
Reading is a critical skill that affects the development of other core skills such as math, as well as self-esteem. Dyslexia is a learning disability characterized by a difficulty to read or interpret words, letters, and symbols according to their shapes5. Dyslexia is not tied to IQ6 and students with dyslexia perform well as long as they get the accommodations they require. In order to qualify for these accommodations, students must undergo a long detection process, testing, and finally receive a diagnosis by a school psychologist offered by their U.S. school system. It is not uncommon for this process to take years, delaying diagnosis and missing critical years of learning and growth, causing the child to fall further and further behind. If a child struggles to read in third grade they are at a higher risk of high school dropout. However, currently only 25% of children with dyslexia are actually detected by that age7. Our research aims to develop methods of automating the early detection process, taking the burden off of teachers and removing detection bias based on race or socioeconomic status.
The Study
Study Procedures
We have been approved by the IRB to do a study to collect a more robust data set for this project. The old data set was collected through an open call to parents, and was very messy, as we did not require a standardized paragraph (all of the students wrote different things), or a standardized sample material (they all wrote on different types of paper with different writing utensils, making image processing difficult). The goal of this study is to collect at least 50 samples from students with diagnosed dyslexia, and at least 100 samples from students without dyslexia (or with un-diagnosed dyslexia).
Parents of children K-6th grade are asked to instruct their children to perform a variety of handwriting tasks on a provided handwriting sample collection form.
- Reading a list of words out loud and having their child write them down (these are Frye words, or sight words, commonly used to detect reading problems).
- Reading a paragraph out loud and having their child write down the paragraph on the sample.
- Timing their child while the child brainstorms and writes a short story, provided a story starter (a sentence) on the sample.
An optional lesson plan has also been provided. This lesson plan covers the importance of machine learning and data security, and describes to the children why our research on their handwriting is important.
One part of the lesson plan: Google QuickDraw! Students can see how Google “learns” what image they are drawing. It is an easy and intuitive way to understand machine learning. This data collection form contains three main sections, each of which has a different purpose.
Interested in contributing to the project?
We are part of the Indiana University Bloomington School of Informatics, Computing and Engineering working on a research project, the purpose of which is to analyze features of student handwriting to improve machine learning and computer vision algorithms. We would like to invite you and your child (K-6th grade) to participate in this project by uploading a handwriting sample using the attached instructions. The time commitment is ~20 minutes, or ~45 minutes if you choose to give your child the optional lesson plan on data security and handwriting recognition. If you choose to participate, you can access the materials and the online form here, and you will be asked to read an informed consent form. We would like you to print the handwriting collection form, but if you don’t have access to a printer we will accept normal paper. You will be compensated with a $10 Amazon gift card for your time. If you have any questions, please contact Katie Spoon at kspoon@iu.edu. We appreciate your time!
Current State of the Project
All of our current results have been calculated using a preliminary data set, however once we have completed collecting the new, more robust data set, we will re-run the neural network experiments on the new data. Before the end of the spring semester, we will be releasing the code and data set, along with a preliminary web application that parents and teachers could potentially use to test a handwriting sample for indications of reading problems.
One area that we will try to focus on moving forward is explaining the results of the neural network. Neural networks act as a “black box”, meaning they take in information and produce an output (in our case, whether or not a student could potentially have dyslexia), without any explanation as to how it reached that decision. The next phase of the project is to use data visualization techniques to try to figure out what is happening “under the hood”, as well as searching for any bias or strange methods in the network.
Contact
Contact Katie Spoon at kspoon@iu.edu with any questions or comments about the project!
People:
Learn more about the project:
- Watch this 6-minute video created for the NCWIT (National Center for Women in Technology) award submission
- Read this article on the School of Informatics, Computing and Engineering website
- Check out the poster:
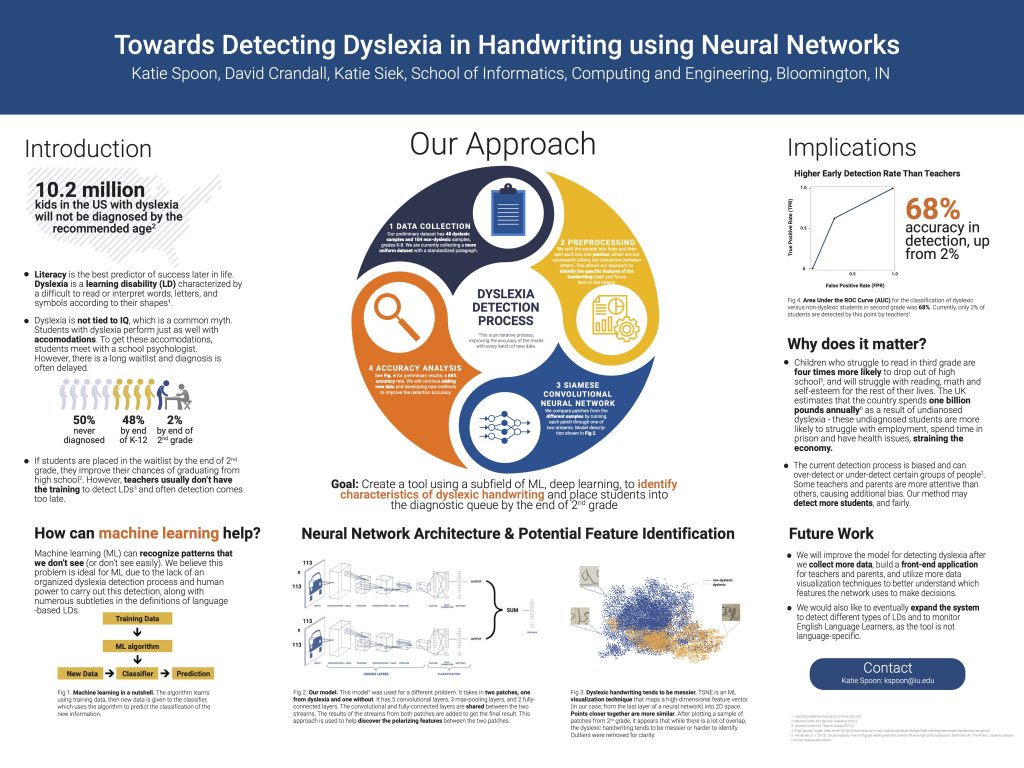
Additional Information
Learn more about dyslexia:
- The State of Learning Disabilities: Understanding the 1 in 5
- Decoding Dyslexia, a grassroots parental advocacy organization
- National Center on Improving Literacy (NCIL)
- Dyslexia FAQs
References
- National Center for Learning Disabilities. The state of LD: Understanding the 1 in 5. https://www.ncld.org/archives/blog/the-state-of-ld-understanding-the-1-in-5, 2017.
- Donald J. Hernandez. Double jeopardy: How third-grade reading skills and poverty influence high school graduation. Annie E. Casey Foundation, 2011.
- Ted Tolfree, Derrik Jones, Martin Raby and Jean Gross. The long-term costs of literacy difficulties. KPMG Foundation, 2006.
- Glaser D. Wilcox, D. D. Walsh, K. What education schools aren’t teaching about reading and what elementary teachers aren’t learning. National Council on Teacher Quality, 2006.
- Learning Disabilities Association of America. Dyslexia. https://ldaamerica.org/types-of-learningdisabilities/dyslexia/
- Hiroko Tanaka, Jessica M. Black, Leanne M. Stanley, Shelli R. Kesler, Allan L. Reiss, Charles Hulme, John D. E. Gabrieli, Fumiko Hoeft and Susan Whitfield-Gabrieli. The brain basis of the phonological deficit in dyslexia is independent of IQ. Psychological Science, 22:1442–1451, 2011.
- National Center for Learning Disabilities. Identifying struggling students. https://www.ncld.org/identifying-struggling-students