- pdf: http://papers.nips.cc/paper/8570-meta-reinforced-synthetic-data-for-one-shot-fine-grained-visual-recognition.pdf
- code: https://github.com/apple2373/MetaIRNet
- pdf of slides: https://drive.google.com/file/d/1YQtKEn4ySVqsMMU66dS1JvVhDWpO4Qqz/view?usp=sharing
- poster: http://vision.soic.indiana.edu/wp/wp-content/uploads/satoshi-poster-edited2.pdf
@inproceedings{metasatoshi19,
author={Satoshi Tsutsui, Yanwei Fu, David Crandall},
booktitle = {Advances in Neural Information Processing Systems (NeurIPS)},
title = {{Meta-Reinforced Synthetic Data for One-Shot Fine-Grained Visual Recognition}},
year = {2019}
}
We introduce an effective way to employ an ImageNet-pre-trained image generator for the purpose of improving fine-grained one-shot classification when data is scarce. As a way to fine-tune the pre-trained generator, our pilot study finds that adjusting only scale and shift parameters in batch normalization can produce a visually realistic images. This way works with a single image making the method less dependent on the number of available images. Furthermore, although naively adding the generated images into the training set does not improve the performance, we show that it can improve the performance if we properly mix the generated images with the original image. In order to learn the parameters of this mixing, we adapt a meta-learning framework. We implement this idea and demonstrate a consistent and significant improvement over several classifiers on two fine-grained benchmark datasets.
Framework
Our Meta Image Reinforcing Network (MetaIRNet) has two modules: an image fusion network, and a one-shot classification network. The image fusion network reinforces generated images to try to make them beneficial for the one-shot classifier, while the one-shot classifier learns representations that are suitable to classify unseen examples with few examples. Both networks are trained by end-to-end, so the loss back-propagates from classifier to the fusion network.
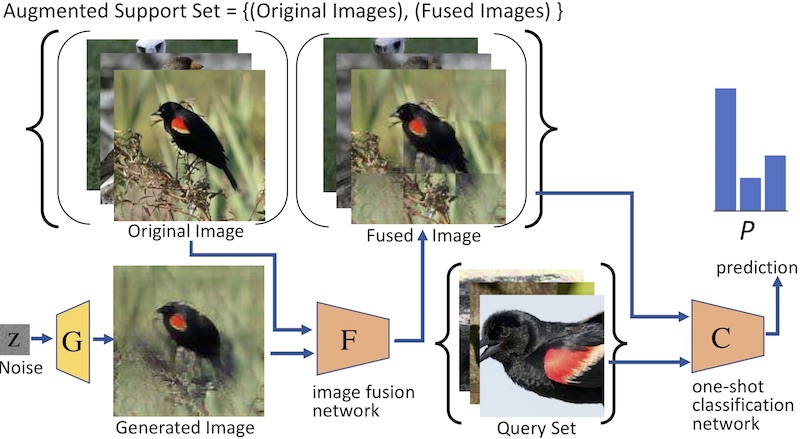
Fine-tune BigGAN with a single image
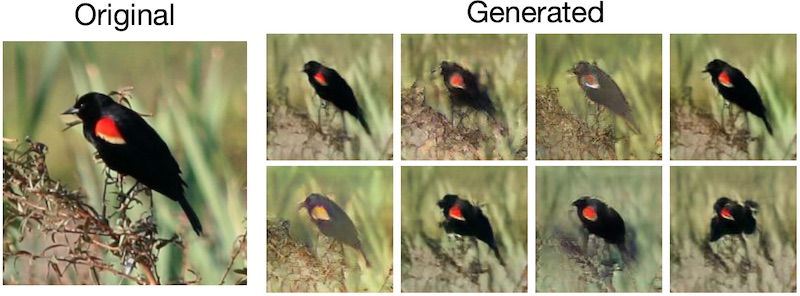
Reinforced Images by MetaIRNet
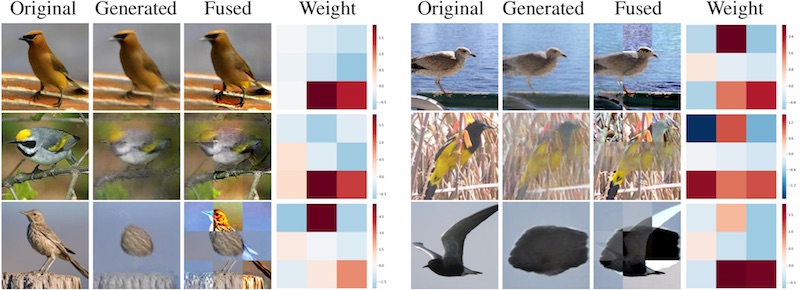